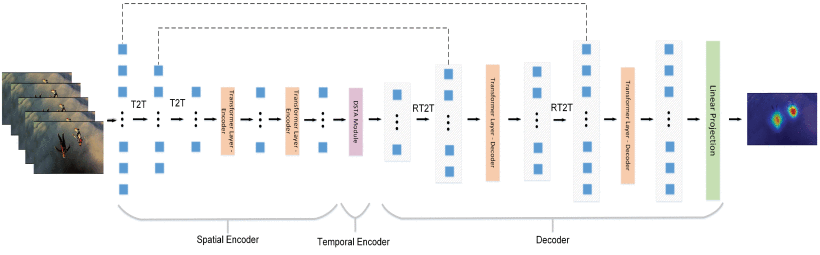
TempVST: Our Latest Video Saliency Prediction Model Published in IEEE Access
We are thrilled to share the exciting news that our paper, “The Visual Saliency Transformer Goes Temporal: TempVST for Video Saliency Prediction,” has been accepted for publication in IEEE Access. In this work, an innovative model designed to enhance video saliency prediction by extending the capabilities of the Visual Saliency Transformer (VST) is introduced.
Video saliency prediction refers to the process of identifying which parts of a video are likely to capture the most attention from viewers. It’s a crucial aspect of video analysis, as via this process, the elements that draw focus over time in a video are identified.
Our new model, TempVST, not only builds on the strengths of the VST for static image analysis but also introduces a temporal module that allows it to capture how attention shifts across frames in a video. This makes it possible to predict what parts of a video sequence will be most visually engaging as the video progresses.
Our approach represents a significant advancement in the field of video saliency prediction, offering a robust solution that transitions architectural models from the image domain to the more complex video domain. We are excited about the potential applications of TempVST and the contribution it will make to the broader research community.
The full publication can be accessed via the IEEE portal.
Citation: Lazaridis, N., Georgiadis, K., Kalaganis, F., Kordopatis-Zilos, G., Papadopoulos, S., Nikolopoulos, S., & Kompatsiaris, I. (2024). The Visual Saliency Transformer Goes Temporal: TempVST for Video Saliency Prediction. IEEE Access. https://doi.org/10.1109/ACCESS.2024.3436585