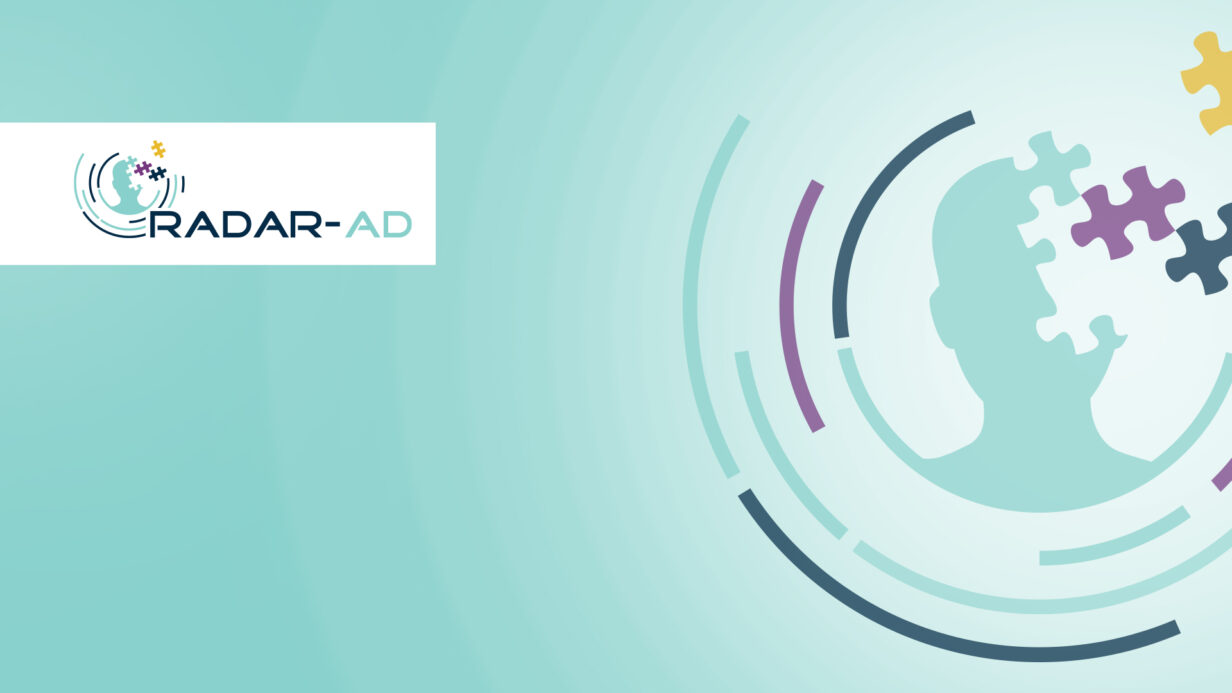
RADAR-AD study findings published in Alzheimer’s Research & Therapy
The results of the RADAR-AD study, which explored the potential of digital technologies to enhance the assessment of Alzheimer’s disease (AD), have been published in Alzheimer’s Research & Therapy.
Led by Fraunhofer, this publication marks a key milestone for the consortium, presenting the first analysis results from the entire set of features obtained from the Remote Monitoring Technologies (RMTs) used by participants over the course of eight weeks. The study involved 229 participants across four stages of cognitive decline: healthy controls, participants with preclinical AD, prodromal AD, and mild-to-moderate AD, from 13 European sites. The findings provide valuable insights into the potential of RMTs to distinguish between different stages of cognitive decline and effectively monitor the progression of Alzheimer’s disease.
The publication presents the analysis of the features obtained from six RMTs, namely: Fitbit Charge 3 wearable: (tracking physical activity, heart rate, sleep patterns), Axivity AX3 wearble (monitoring movement patterns, physical activity levels), the Mezurio app (assessing cognitive function through interactive tasks, digital cognitive testing), the Altoida app (evaluating function using augmented reality), Physilog sensors (measuring gait, balance, mobility), and the Banking app – developed by CERTH to assess the ability of managing finances through an application that simulates an ATM.
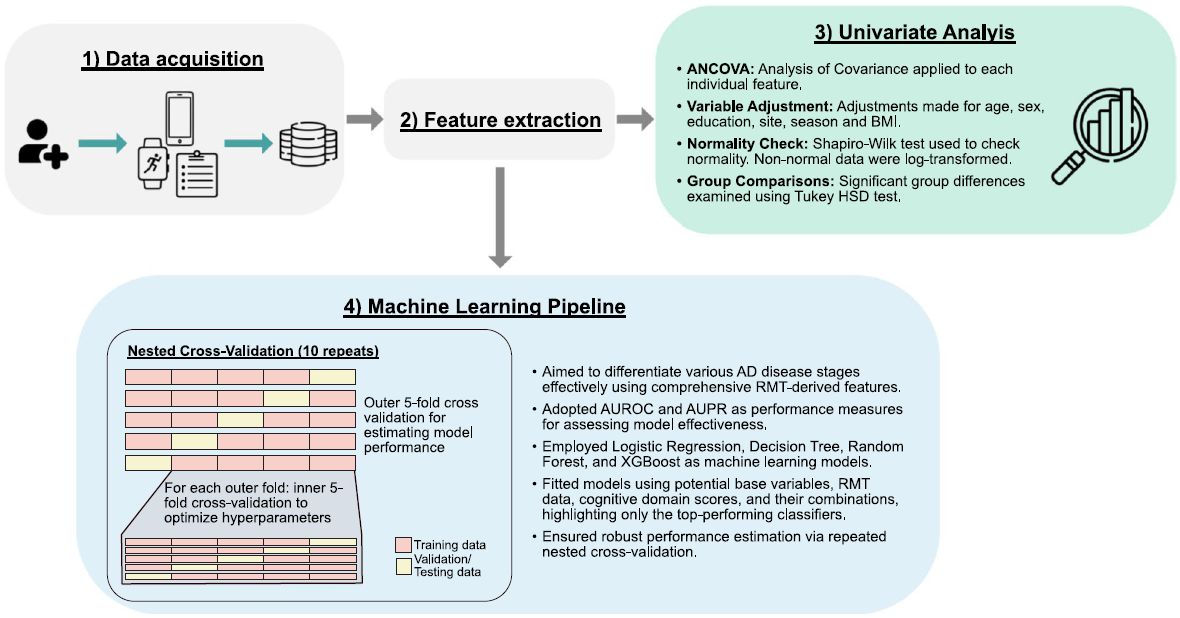
The methodology followed, involved initially a univariate analysis to investigate the existence of prominent features per RMT that could distinguish between the four groups of participants. Several differences could be identified between healthy controls and the prodromal or the more advanced mild-to-moderate group in terms of reduced physical activity (Axivity features), less REM sleep (Fitbit), altered gait patterns (Physilog) and decreased cognitive functioning (Altoida, Banking app).
As the univariate analysis approach does not allow for the identification of complex interactions and patterns, a machine learning pipeline was also used to explore the entire set of features of the RMTs. In the machine learning models, the Axivity wearable proved to be the most effective RMT for differentiating healthy controls from individuals with preclinical Alzheimer’s disease, while the Mezurio app performed exceptionally in distinguishing healthy controls from those with mild-to-moderate AD, despite not showcasing distinguishing features in the univariate analysis.
The study highlights that while certain RMTs performed well, a multi-feature approach is essential for improving classification accuracy. It also emphasizes the challenge of detecting functional differences in the early stages of Alzheimer’s disease while demonstrating the effectiveness of RMTs in identifying individuals in the prodromal or mild-to-moderate stages compared to healthy controls.
Read the full paper: M. Lentzen, S. Vairavan, M. Muurling, V. Alepopoulos, A. Atreya, M. Boada, C. de Boer, P. Conde, J. Curcic, G. Frisoni, S. Galluzzi, M. T. Gjestsen, M. Gkioka, M. Grammatikopoulou, L. Hausner, C. Hinds, I. Lazarou, A. de Mendonça, S. Nikolopoulos, D. Religa, G. Scebba, P. J. Visser, G. Wittenberg, V. A. Narayan, N. Coello, A.K. Brem, D. Aarsland and H. Fröhlich on behalf of RADAR-AD, “RADAR-AD: assessment of multiple remote monitoring technologies for early detection of Alzheimer’s disease,” Alzheimer’s Research & Therapy, Volume 17, Article number 29, (2025), January 2025.